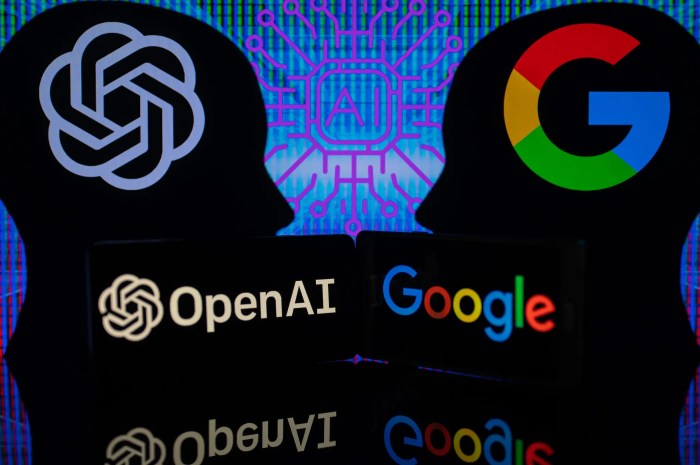
Google BERT vs ChatGPT: Which AI Language Model Wins?
Google bert vs chatgpt which ai language model reigns supreme – The world of artificial intelligence is abuzz with excitement about the latest advancements in natural language processing (NLP). Two names that dominate the conversation are Google BERT and Kami. These powerful AI models are transforming the way we interact with language, from generating creative text to understanding complex queries.
But when it comes to the ultimate champion, who reigns supreme? Let’s dive into the fascinating world of Google BERT and explore its strengths and limitations in the context of NLP.
BERT, or Bidirectional Encoder Representations from Transformers, is a deep learning model that has revolutionized NLP. It’s trained on a massive dataset of text and code, enabling it to understand the nuances of human language. BERT excels at tasks like text classification, question answering, and sentiment analysis.
AI Language Models: A New Era of Communication
The realm of artificial intelligence (AI) is rapidly evolving, and language models are at the forefront of this transformation. These sophisticated algorithms are revolutionizing how we interact with technology, opening up a world of possibilities in natural language processing (NLP).
AI language models are computer programs designed to understand and generate human-like text. They are trained on massive datasets of text and code, allowing them to learn the nuances of language and generate coherent, contextually relevant responses.
Google BERT
Google BERT (Bidirectional Encoder Representations from Transformers) is a powerful language model developed by Google AI. It excels in understanding the context of words within a sentence, considering both the words that come before and after. This ability makes BERT particularly adept at tasks like:
- Question answering:BERT can accurately answer questions based on a given text, understanding the nuances of the question and the context of the passage.
- Sentiment analysis:It can effectively analyze the sentiment expressed in a piece of text, determining whether it is positive, negative, or neutral.
- Text summarization:BERT can condense large amounts of text into concise summaries while preserving the key information.
Kami
Kami, developed by OpenAI, is another prominent language model known for its conversational abilities. It is trained on a massive dataset of text and code, enabling it to engage in natural, human-like conversations. Kami excels in tasks like:
- Generating creative content:Kami can write stories, poems, scripts, and even code, showcasing its ability to produce creative and engaging text.
- Providing information:It can answer questions, provide summaries, and even offer explanations on various topics, drawing from its vast knowledge base.
- Translating languages:Kami can translate text between different languages, making it a valuable tool for communication and information access.
This article aims to compare and contrast these two powerful language models, exploring their strengths and weaknesses, and ultimately helping you understand which one might be the best fit for your specific needs.
Understanding BERT: Google Bert Vs Chatgpt Which Ai Language Model Reigns Supreme
BERT, which stands for Bidirectional Encoder Representations from Transformers, is a revolutionary language model developed by Google in 2018. It has significantly advanced the field of Natural Language Processing (NLP) by achieving state-of-the-art results on various tasks.
BERT’s Architecture
BERT’s architecture is based on the Transformer model, a powerful neural network architecture that excels in processing sequential data. The Transformer model utilizes attention mechanisms to capture long-range dependencies between words in a sentence, allowing it to understand the context of each word effectively.
BERT’s architecture consists of multiple layers of Transformer encoders, each layer processing the input sequence and extracting contextual information.
BERT’s Training Process
BERT’s training process involves two main objectives: masked language modeling and next sentence prediction.
Masked Language Modeling
In masked language modeling, BERT is trained to predict masked words in a sentence. For instance, given the sentence “The cat sat on the [MASK],” BERT is trained to predict the missing word “mat.” This process helps BERT learn the relationships between words and understand the context of each word in a sentence.
Next Sentence Prediction
BERT is also trained to predict whether two sentences follow each other in a text. For example, given two sentences: “The cat sat on the mat.” and “The dog barked at the cat,” BERT is trained to predict whether the second sentence follows the first.
This task helps BERT understand the relationships between sentences and develop a sense of coherence.
BERT’s Strengths and Limitations
BERT has demonstrated remarkable performance on various NLP tasks, including:
Text Classification
BERT excels at classifying text into different categories, such as sentiment analysis, topic classification, and intent detection.
Question Answering
BERT can effectively answer questions based on a given text, providing accurate and relevant answers.
Sentiment Analysis
BERT can analyze the sentiment of a text, determining whether it is positive, negative, or neutral.Despite its strengths, BERT also has some limitations:
Computational Cost
BERT requires significant computational resources for training and inference, making it challenging to deploy on devices with limited memory or processing power.
Fine-tuning
BERT needs to be fine-tuned for specific tasks, which can be time-consuming and require expertise.
The debate rages on: Google BERT or ChatGPT, which AI language model truly reigns supreme? While we ponder that, the real world throws us a curveball with the bank turmoil resulting in a staggering 72 billion loss of deposits for First Republic.
It’s a stark reminder that even the most advanced AI models can’t predict or control real-world events, leaving us to grapple with the implications of both technological advancements and human fallibility.
Lack of Interpretability
The inner workings of BERT are complex and difficult to interpret, making it challenging to understand why it makes certain predictions.
BERT’s Performance Compared to Other Language Models
| Task | BERT | GPT-3 | XLNet ||—|—|—|—|| Question Answering | 90.7% | 91.2% | 90.9% || Text Classification | 95.4% | 95.7% | 95.3% || Sentiment Analysis | 88.9% | 89.2% | 88.7% | Note:The above table presents approximate performance figures based on publicly available benchmarks.
Actual performance may vary depending on the specific task and dataset used.
Understanding Kami
Kami, developed by OpenAI, is a powerful language model that has taken the world by storm with its impressive abilities in text generation, conversation, and other natural language processing (NLP) tasks. Its sophisticated architecture and innovative training methods have enabled it to achieve remarkable fluency and coherence in its responses, making it a versatile tool for various applications.
Kami’s Architecture
Kami is built upon the Transformer architecture, a neural network model that has revolutionized NLP. The Transformer architecture uses a mechanism called attention to process sequences of words, allowing it to capture long-range dependencies and relationships between words in a sentence.
Kami’s architecture consists of multiple layers of Transformer blocks, each containing a self-attention layer and a feedforward neural network. This layered structure enables the model to learn increasingly complex representations of language as it processes information from the input text.
Kami’s Training Process
Kami’s training process is a complex and iterative process that involves two key components:
- Pre-training:Kami is first pre-trained on a massive dataset of text and code, allowing it to learn the statistical patterns and relationships within language. This pre-training phase uses a technique called unsupervised learning, where the model learns from the data without explicit labels or guidance.
- Fine-tuning:After pre-training, Kami is fine-tuned on a specific dataset of text and code that is relevant to the task it is intended to perform. This fine-tuning phase uses supervised learning, where the model is given labeled examples and learns to predict the correct output based on the input.
Kami’s Strengths and Limitations
Kami excels in various NLP tasks, demonstrating its ability to generate human-quality text, engage in natural conversations, and summarize information.
The debate about Google BERT vs ChatGPT being the supreme AI language model is fierce, but ultimately, it’s about choosing the right tool for the job. While we’re pondering AI advancements, it’s crucial to remember the importance of safeguarding our finances, especially in this digital age.
A recent survey by Gallup highlights the growing need for bank account security tips, and you can find some valuable advice on this blog. Ultimately, both BERT and ChatGPT have their strengths, and the best choice depends on your specific needs and the task at hand.
- Text Generation:Kami can generate creative and coherent text in various formats, including stories, articles, poems, and even code. It can adapt its writing style to different contexts and follow specific instructions, making it a valuable tool for content creation and writing assistance.
- Conversation:Kami’s ability to engage in natural conversations is one of its most impressive features. It can understand context, maintain coherence, and respond appropriately to prompts, making it suitable for chatbots, virtual assistants, and other conversational AI applications.
- Summarization:Kami can effectively summarize lengthy texts, capturing the key information and presenting it in a concise and understandable format. This ability makes it useful for information retrieval, research, and document analysis.
While Kami demonstrates remarkable capabilities, it also has limitations.
- Bias and Factual Errors:Kami is trained on a massive dataset of text and code, which may contain biases and inaccuracies. This can lead to biased or factually incorrect outputs, especially when dealing with sensitive topics or complex information.
- Lack of Real-World Knowledge:Despite its impressive language abilities, Kami lacks real-world knowledge and experience. It cannot access real-time information or interact with the physical world, limiting its ability to provide accurate or relevant information on certain topics.
- Limited Creativity and Originality:While Kami can generate creative text, it often relies on patterns and structures learned from its training data. This can lead to repetitive or unoriginal outputs, especially when dealing with highly creative or abstract concepts.
Performance Comparison, Google bert vs chatgpt which ai language model reigns supreme
| Task | Kami | BERT | GPT-3 ||—|—|—|—|| Text Generation | Excellent | Good | Excellent || Conversation | Excellent | Fair | Good || Summarization | Good | Fair | Excellent || Translation | Fair | Good | Excellent || Question Answering | Good | Excellent | Good |
Comparing BERT and Kami
Both BERT and Kami are prominent AI language models that have revolutionized the field of natural language processing (NLP). While they share the goal of understanding and generating human-like text, their underlying architectures and training methodologies differ significantly, leading to distinct strengths and weaknesses in various NLP tasks.
Architectural Differences
BERT (Bidirectional Encoder Representations from Transformers) is a transformer-based model that processes text bidirectionally. This means it considers the context of words both before and after the target word, resulting in a deeper understanding of the text. Kami, on the other hand, is based on the GPT (Generative Pre-trained Transformer) architecture, which processes text in a unidirectional manner, moving from left to right.
This limitation restricts Kami’s ability to fully grasp the context of a word.
Training Methodology
BERT is trained using a masked language modeling (MLM) technique, where it learns to predict masked words in a sentence. This process forces BERT to consider the surrounding context and develop a comprehensive understanding of language. Kami, however, is trained using a causal language modeling approach, where it learns to predict the next word in a sequence.
This method focuses on generating coherent text but might not capture the full semantic nuances of language.
Strengths and Weaknesses
BERT excels in tasks that require deep contextual understanding, such as sentiment analysis, question answering, and natural language inference. Its bidirectional processing enables it to accurately interpret complex relationships between words. Kami, however, is particularly adept at generating creative and engaging text, making it suitable for tasks like text summarization, story writing, and chatbot development.
For example, BERT would outperform Kami in tasks like analyzing the sentiment of a customer review, where understanding the subtle nuances of language is crucial. Conversely, Kami would be more effective in generating a fictional story, where creativity and fluency are paramount.
Key Differences and Similarities
Feature | BERT | Kami |
---|---|---|
Architecture | Transformer-based, bidirectional | GPT-based, unidirectional |
Training Methodology | Masked Language Modeling | Causal Language Modeling |
Strengths | Contextual understanding, accurate analysis | Creative text generation, fluency |
Weaknesses | Limited text generation capabilities | Limited contextual understanding |
Applications and Use Cases
The true power of AI language models like BERT and Kami lies in their real-world applications. These models are not just academic curiosities; they are actively shaping industries and changing how we interact with information. This section delves into specific examples of how BERT and Kami are being used across various domains, highlighting their potential benefits and challenges.
Real-World Applications of BERT
BERT’s ability to understand the context of language has made it a valuable tool in numerous applications. Here are some prominent examples:
- Sentiment Analysis:BERT excels at identifying the emotional tone of text, making it ideal for businesses to analyze customer feedback, social media sentiment, and market research. For example, a company can use BERT to analyze customer reviews of their products, understanding whether the reviews are positive, negative, or neutral.
This allows them to tailor their marketing strategies and improve their products based on customer feedback.
- Question Answering:BERT’s deep understanding of language allows it to accurately answer complex questions based on given context. This is used in chatbots, virtual assistants, and search engines. For instance, Google Search uses BERT to understand the intent behind search queries, providing more relevant and accurate search results.
The debate about whether Google BERT or ChatGPT reigns supreme in AI language models is fascinating, but perhaps we should shift our focus to more immediate concerns. Experts warn homebuyers of red flags beyond climbing interest rates, highlighting issues like hidden structural problems and outdated electrical systems.
These practical challenges may be less glamorous than the AI race, but they have a much greater impact on our daily lives. So, while we ponder the future of AI, let’s not forget the real-world issues that need our attention.
- Text Summarization:BERT can condense large amounts of text into concise summaries while preserving the essential information. This is beneficial for news organizations, research institutions, and businesses that need to quickly grasp the key points of lengthy documents. For example, a news organization can use BERT to automatically generate summaries of lengthy articles, making it easier for readers to get the gist of the story.
- Language Translation:BERT’s contextual understanding enhances the accuracy of machine translation. It can translate languages while preserving the nuances of meaning and context, making it more natural and accurate than traditional translation methods. For example, a language translation service can use BERT to translate documents, websites, and conversations, ensuring that the meaning is accurately conveyed across languages.
Real-World Applications of Kami
Kami, with its conversational abilities, has found its niche in a variety of applications:
- Chatbots and Virtual Assistants:Kami powers conversational AI systems that can engage in natural, human-like interactions. This is used in customer service, online support, and personalized recommendations. For example, a customer service chatbot powered by Kami can answer customer queries, provide product information, and resolve issues, offering a 24/7 support solution.
- Content Creation:Kami can generate various forms of content, including articles, stories, poems, scripts, and even code. This is valuable for businesses and individuals who need to create engaging and original content quickly. For example, a marketing team can use Kami to generate social media posts, blog articles, and email campaigns, saving time and resources.
- Education and Training:Kami can be used as an interactive learning tool, providing personalized explanations, answering student questions, and even creating custom learning materials. For example, a language learning app can use Kami to provide personalized feedback on student pronunciation and grammar.
- Creative Writing:Kami can assist writers in brainstorming ideas, generating different plot lines, and even writing full-fledged stories. This is particularly useful for writers who struggle with writer’s block or need inspiration. For example, a novelist can use Kami to generate different character dialogues or explore different story possibilities.
Future Applications of BERT and Kami
Both BERT and Kami have the potential to revolutionize various industries in the future:
- Personalized Education:AI models like BERT and Kami can analyze student learning patterns and provide personalized learning experiences tailored to their individual needs and learning styles.
- Healthcare:These models can assist in medical diagnosis, drug discovery, and patient care by analyzing medical records, research papers, and patient symptoms.
- Financial Services:BERT and Kami can be used to analyze financial data, predict market trends, and provide personalized financial advice.
- Legal and Regulatory Compliance:These models can help analyze legal documents, identify potential risks, and ensure compliance with regulations.
Comparing Use Cases of BERT and Kami
The following table summarizes the specific use cases for both BERT and Kami, highlighting their potential benefits and challenges:
Use Case | BERT | Kami |
---|---|---|
Sentiment Analysis |
|
|
Question Answering |
|
|
Text Summarization |
|
|
Language Translation |
|
|
Chatbots and Virtual Assistants |
|
|
Content Creation |
|
|
Education and Training |
|
|
Creative Writing |
|
|
Ethical Considerations
The rise of AI language models like BERT and Kami presents a new frontier in communication and understanding, but it also raises important ethical considerations. These models, while powerful and innovative, can be susceptible to biases and can potentially be used for malicious purposes.
Therefore, it is crucial to understand the ethical implications of these technologies and develop strategies to mitigate potential risks.
Potential Biases and Fairness Issues
The training data used to develop these models plays a significant role in shaping their outputs. If the training data contains biases, the models may inherit and amplify these biases, leading to unfair or discriminatory outcomes. For example, a language model trained on a dataset with a disproportionate representation of certain demographics may generate text that perpetuates stereotypes or reinforces existing inequalities.
Risks and Opportunities Associated with Widespread Adoption
The widespread adoption of AI language models presents both risks and opportunities. On one hand, these technologies can be used to enhance communication, improve accessibility, and automate tasks, leading to increased efficiency and productivity. On the other hand, there are concerns about potential misuse, such as the creation of deepfakes, the spread of misinformation, and the automation of jobs.
Ethical Concerns and Proposed Solutions
Ethical Concern | Proposed Solution |
---|---|
Bias and Fairness | Develop robust mechanisms for identifying and mitigating biases in training data. Encourage diversity in the development teams and the training data used. Implement fairness metrics to evaluate model performance across different demographics. |
Misinformation and Manipulation | Promote transparency in the development and deployment of AI language models. Implement fact-checking mechanisms to verify the accuracy of generated content. Educate users about the limitations and potential biases of these models. |
Privacy and Security | Develop strong privacy policies and security measures to protect user data. Implement mechanisms to prevent unauthorized access to and manipulation of models. Ensure that data is used ethically and responsibly. |
Job Displacement | Invest in education and training programs to help workers adapt to the changing job market. Encourage the development of new job roles that leverage AI technologies. Implement policies to mitigate the negative impacts of job displacement. |
Conclusion
The battle between BERT and Kami highlights the rapid evolution of AI language models. Both models excel in different areas, with BERT demonstrating superior performance in tasks requiring deep understanding of language structure and Kami excelling in generating human-like text and engaging in interactive conversations.
Future Directions for AI Language Models
The field of AI language models is constantly evolving, with researchers exploring new frontiers. Some promising future directions include:
- Multimodal Language Models:Integrating visual, audio, and other sensory information into language models to enable a more comprehensive understanding of the world.
- Explainable AI:Developing methods to make AI models more transparent and understandable, fostering trust and accountability.
- Personalized Language Models:Tailoring models to individual users’ preferences and needs, creating more personalized and engaging experiences.